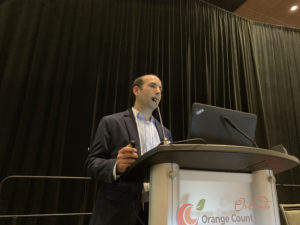
Eran Halperin, Ph.D.
Artificial intelligence (AI) and machine learning are no longer new terms in the language of anesthesiology. Yet it is allowing anesthesiologists to make new strides in everyday practice and patient care. A look at the challenges and caveats of AI in anesthesiology was the focus of Saturday’s session “Artificial Intelligence in Perioperative Medicine: Moving from Big Data to Smart Data.”
The volume of health-related data generated in the perioperative setting is growing exponentially. Today, new analytical techniques are available to augment the value of these data. Specifically, the use of AI and machine learning approaches has potential to radically change our understanding of patients, risk trajectory and clinical management. Eran Halperin, Ph.D., of the University of California, Los Angeles opened the session by summarizing what we know, what works and what remains to be seen on the topic.
“Machine learning creates algorithms that can learn from data and make predictions on new data by finding patterns in high-dimensional data, sifting through large volumes of data and predicting and classifying models,” Dr. Halperin said. “AI’s impact is already here. It can’t yet replace the clinician’s work, but it may help where big data is available.”
During the session, speakers addressed:
- The basics of machine learning and AI techniques applied to anesthesiology and perioperative medicine
- Potential applications of machine learning using multi-modal data sources in the perioperative setting
- Practical approaches focusing on machine learning to predict post-induction hypotension.
According to Dr. Halperin, AI and machine learning are especially helpful in the area of genomics, where clinicians can apply large amounts of available data to find a small number of biomarkers. Conversely, challenges and caveats do exist, Dr. Halperin said, including in the areas of outfitting/leakage, confounders, interpretability, sparsity of the data, measuring performance, implementation and phenotyping.
The overfitting challenge, for example, is that the data are being memorized instead of learned and there is bad generalization. For data leakage, training data “leaks” to test data, results are too good to be true and the data is difficult to calculate until deployment.
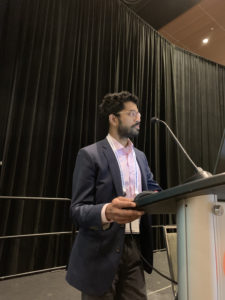
Samir Kendale, M.D., FASA
The benefits of AI are valuable at the patient level, he said. For example, using perioperative features, AI can predict surgery outcomes, specifically predicting mortality and predicting acute kidney injury (AKI) within admission.
“Using wearable features, AI can even predict whether a person will be more depressed within the next two weeks post-surgery,” Dr. Halperin said.
AI provides anesthesiologists with “reinforcement learning,” he said. “The prediction interacts with the clinician’s actions.”
Samir Kendale, M.D., FASA, an Assistant Professor of Anesthesiology at New York University Langone Health, was among the session speakers who addressed machine learning for postinduction hypotension as a case example for demonstrating the machine learning model from a clinical perspective. Dr. Kendale urged physicians to be centrally involved in the process of machine learning development, and that doing so requires an understanding of the basics. This includes analyzing critical machine learning reports and manuscripts and identifying the various types of data available from EMRs and how they can be leveraged for reporting in the perioperative environment.
“After years of the dream of AI being fed to us through countless science fiction books and movies (which were and continue to be an enduring presence in my life), we are at a major technological turning point where those dreams have a real potential of becoming reality,” Dr. Kendale said.
To study postinduction hypotension, the clinician needs a brain, a computer and lots of data, he said. When evaluating that data, Dr. Kendale urged others to consider what you have, what you need, where you mine the data and whether you trust it. Data leakage is one factor that anesthesiologists must consider. Sometimes, for example, data leakage can produce information that leads to an excessively optimist prediction. Therefore, internal and external validation of the data is critical
Ultimately, AI and machine learning require broad collaboration between a number of sources prior to leveraging it for clinical application, Dr. Kendale said. These resources must include hospital administration, the hospital IT department, computer scientists, biomedical engineers and EHRs.
Dr. Kendale reminded attendees that it’s important to assess the pitfalls of machine learning in order to critically appraise the literature. Still, it’s a topic that’s gaining in interest and clinical application.
“We aren’t nearly there yet in the field of anesthesiology, of course–and considering the wide range of obstacles, it will still probably be a number of years before it remotely resembles the fictional representations,” Dr. Kendale said. “But I couldn’t resist becoming part of this exciting journey in these very early stages.”
Return to Archive Index